Breaking Boundaries: Aliaga and team advance urban-related content generation using 100 GPUs
08-04-2023
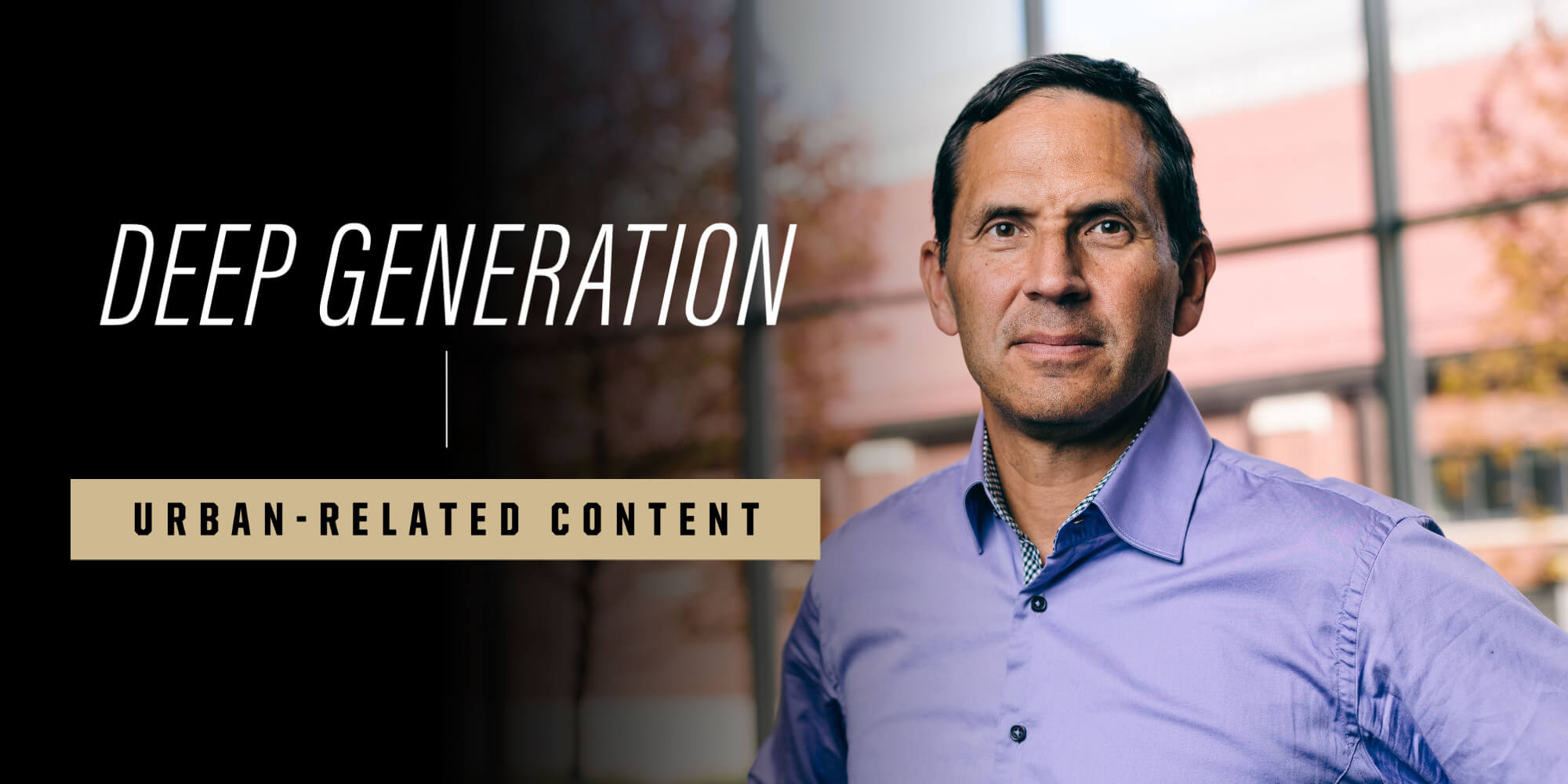
Associate Professor Daniel Aliaga
The research pushed the boundaries of what is possible in generating urban-related content. The utilization of the GPUs achieved unprecedented milestones to significantly contribute to various fields, from computer science to ecological sciences.
In a multidisciplinary endeavor that has far-reaching implications for computer science, urban planning, digital urban forestry, ecological sciences, and related domains, a team led by Daniel Aliaga, associate professor of computer science, has leveraged the power of 100 of the Rosen Center for Advanced Computing (RCAC)’s GPUs to advance the field of deep generation of urban-related content.
Aliaga, with PhD students Adnan Firoze, Liu He, Aocheng Li, and Nikhil Makkar, used the Gilbreth community cluster GPUs to create urban representations for 330 cities across the United States, with a significant emphasis on sustainability and the cities of the future.
The GPUs on RCAC’s Gilbreth community cluster were added as part of recent investments to better support researchers in the fields of AI and machine learning.
“The availability of the GPUs enabled us to improve algorithm development as well as run the model to enable creation of the results,” said Aliaga.
Aliaga and his team embarked on three interlinked projects using the computational power of the GPUs: urban forestry, urban layouts, and historical urban layouts.
“Our research has pushed the boundaries of what is possible in generating urban-related content. The utilization of the RCAC GPUs has allowed us to achieve unprecedented milestones and significantly contribute to various fields, from computer science to ecological sciences.”
Daniel Aliaga, associate professor of computer science
Urban Forestry
The team, together with CS faculty Benes and Yeh and with researchers from the Purdue Institute for Digital Forestry of which Aliaga is a member, used the RCAC GPUs to detect trees in target cities. This resulted in precise localization of 273 million public and private trees throughout 8.2 million acres of urban space. The first-of-its-kind, the dataset computed via automation instead of traditional survey and extrapolation methods.
The GPUs helped to explore hyperparameters and train the underlying models. Once trained, the actual inference process from satellite imagery was relatively fast. Evaluation tests demonstrated the accuracy was in the 85-97% range.
Tree Instance Segmentation with Temporal Contour Graph.
Firoze, C. Wingren, R. Yeh, B. Benes, D. Aliaga, IEEE Computer Vision and Pattern Recognition (CVPR), 2023
Urban Tree Generator: Spatio-Temporal and Generative Deep Learning for Urban Tree Localization and Modeling.
Firoze, B. Benes, D. Aliaga, The Visual Computing Journal (TVCJ), 2022
Urban Layouts
The researchers, together with collaborators from University of Texas at Austin and Prof. Shan from Civil Engineering at Purdue, also used the GPUs to generate urban layouts from sparse incomplete data for use in large-scale digital urban planning applications. In particular, the GPUs enabled the team to explore hyperparameters including clustering and sampling strategies to understand the huge design space.
The researchers arrived at a trained deep engine using only 1-3% of the urban layout information of a city (such as building locations, sizes and heights) that was able to generate the entire urban layout with only a few meters (or percent) of error.
The GPUs were used to optimize more than one billion parameters, using approximately 100 hours wall time, resulting in position errors of less than two meters, area errors of less than 20 square meters, height errors of less than half a meter, and almost zero building count error for several million buildings nationwide.
GlobalMapper: Arbitrary-Shaped Urban Layout Generation.He, D. Aliaga, IEEE Conference on Computer Vision (ICCV), 2023
Deep Learning based Urban Morphology for City-scale Environmental Modeling.
Patel, R. Kalyanam, L. He, D. Aliaga, D. Niyogi. Proceedings of the National Academy of Sciences (PNAS) Nexus, 2023
Generative Building Feature Estimation from Satellite Images.
He, J. Shan, D. Aliaga, IEEE Transactions on Geoscience and Remote Sensing (TGRS), 2023
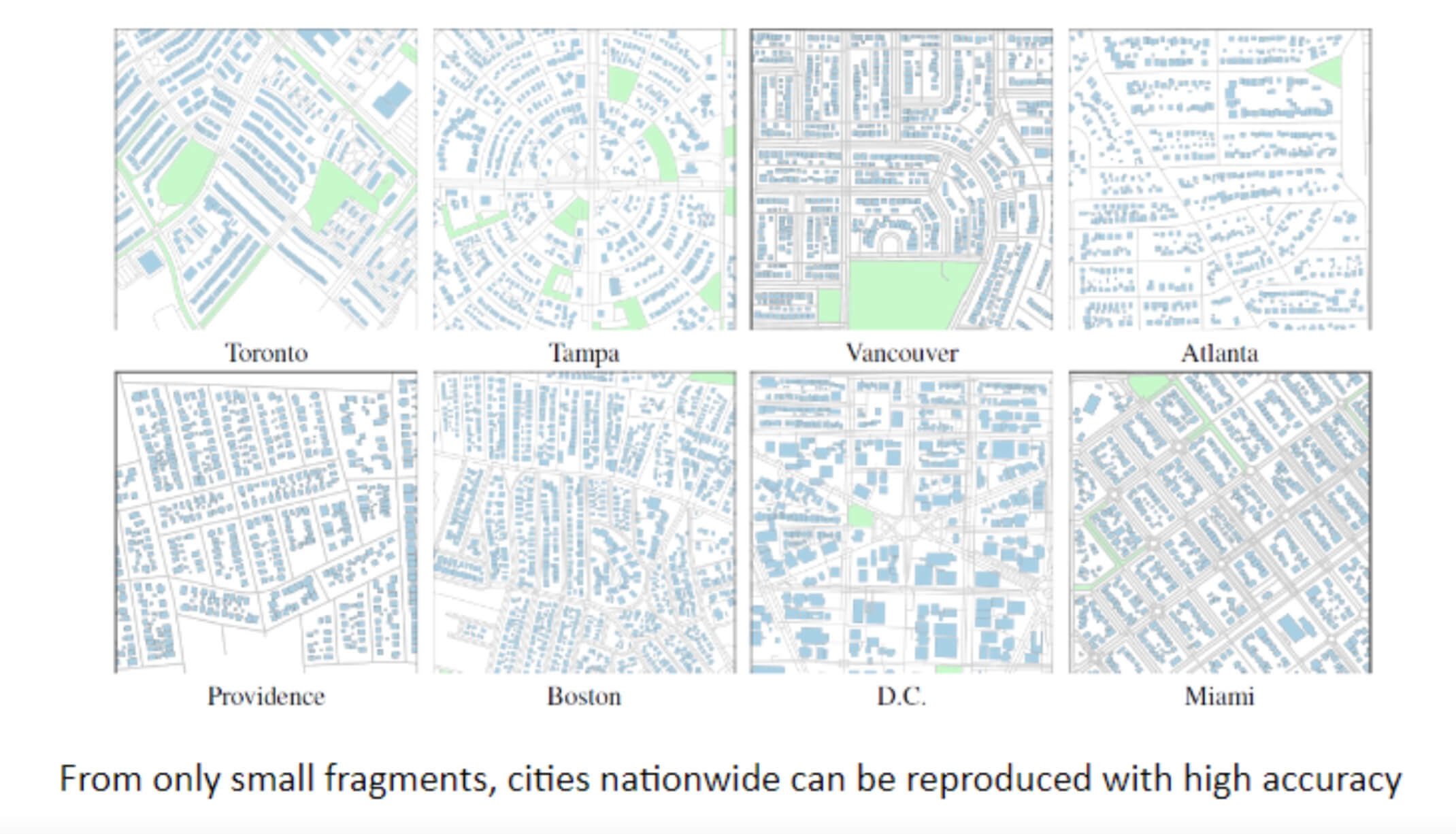
Historical Urban Layouts
The team, in collaboration with the ROSETTA group at Purdue and archaeologists from Vanderbilt University, Brown University, and Mimar Sinan Fine Arts University, is also using the GPUs to help in-progress research of pluralistic image completion and infilling which is useful for archaeological sites, among other applications. Unlike a current city, an ancient archaeological site only has a small percentage of the site remaining. However, this is a similar inference setup, so the same methodology can be applied. Initial results from sites in Peru, Greece, and Turkey are quite promising.
Guided Pluralistic Building Contour Completion.
Zhang, X., Ma, W., Varinlioglu, G., Rauh, N., He, L., & Aliaga, D., The Visual Computer (TVCJ), 2022.
Unprecedented milestones
Aliaga expressed his excitement over the accomplishments of the team, "Our research has pushed the boundaries of what is possible in generating urban-related content.”
He added, “The utilization of the RCAC GPUs has allowed us to achieve unprecedented milestones and significantly contribute to various fields, from computer science to ecological sciences."
“This use case germinated in a discussion at the RCAC Digital Twins symposium where both Daniel Aliaga and Songlin Fei (director of Purdue Institute for Digital Forestry) presented on these three applications and their growing computational needs,” said RCAC research scientist Rajesh Kalyanam.
“It coincided with our expansion of Gilbreth and seemed like the perfect opportunity to explore the possibilities of researchers having access to this unprecedented scale of GPU acceleration at Purdue University.”
About Rosen Center for Advanced Computing
RCAC provides advanced computational resources and services to support Purdue faculty and staff researchers. We also conduct our own research and development to enhance the capabilities of these resources.
About the Department of Computer Science at Purdue University
Founded in 1962, the Department of Computer Science was created to be an innovative base of knowledge in the emerging field of computing as the first degree-awarding program in the United States. The department continues to advance the computer science industry through research. US News & Reports ranks Purdue CS #20 and #16 overall in graduate and undergraduate programs respectively, seventh in cybersecurity, 10th in software engineering, 13th in programming languages, data analytics, and computer systems, and 19th in artificial intelligence. Graduates of the program are able to solve complex and challenging problems in many fields. Our consistent success in an ever-changing landscape is reflected in the record undergraduate enrollment, increased faculty hiring, innovative research projects, and the creation of new academic programs. The increasing centrality of computer science in academic disciplines and society, and new research activities - centered around data science, artificial intelligence, programming languages, theoretical computer science, machine learning, and cybersecurity - are the future focus of the department. cs.purdue.edu
Media contact: Emily Kinsell, emily@purdue.edu
Source: Daniel Aliaga, aliaga@purdue.edu
Parts of this article originally appeared on RCAC.