Paul Valiant
Associate Professor of Computer Science
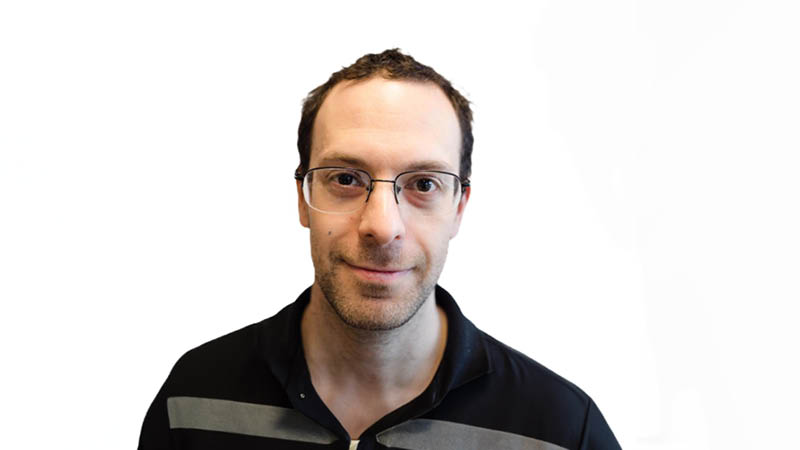
My algorithmic research focuses on sublinear algorithms on big data and related statistics. One branch of this research aims at illuminating the "unseen" portion of a probability distribution - what does the data about customers that visited a website this month enable us to say about the set of customers that did not visit the website? While this entire line of research aims to glean as much value from limited or expensive data as possible, in some cases … ↓More
Joined department: Fall 2021
Research Areas
- Artificial Intelligence, Machine Learning, and Natural Language Processing
- Theory of Computing, Algorithms, and Quantum Computing
My algorithmic research focuses on sublinear algorithms on big data and related statistics. One branch of this research aims at illuminating the "unseen" portion of a probability distribution - what does the data about customers that visited a website this month enable us to say about the set of customers that did not visit the website? While this entire line of research aims to glean as much value from limited or expensive data as possible, in some cases the algorithms have achieved an unusually high bar: "instance optimal" algorithms, general-purpose algorithms that are competitive on each instance even compared to an algorithm custom-designed for that particular instance. Complementing and reinforcing all this work is a focus on developing matching lower bounds; algorithmic lower bounds typically rely on rather different techniques than the algorithms themselves, yet provide an invaluable source of illumination for future algorithmic work in the area.